GeoSpatial Miydi AI Framework
Miydi Maps accelerates AI-based geospatial or mapping projects by offering pre-organized, high-resolution open-source data at the census tract level.
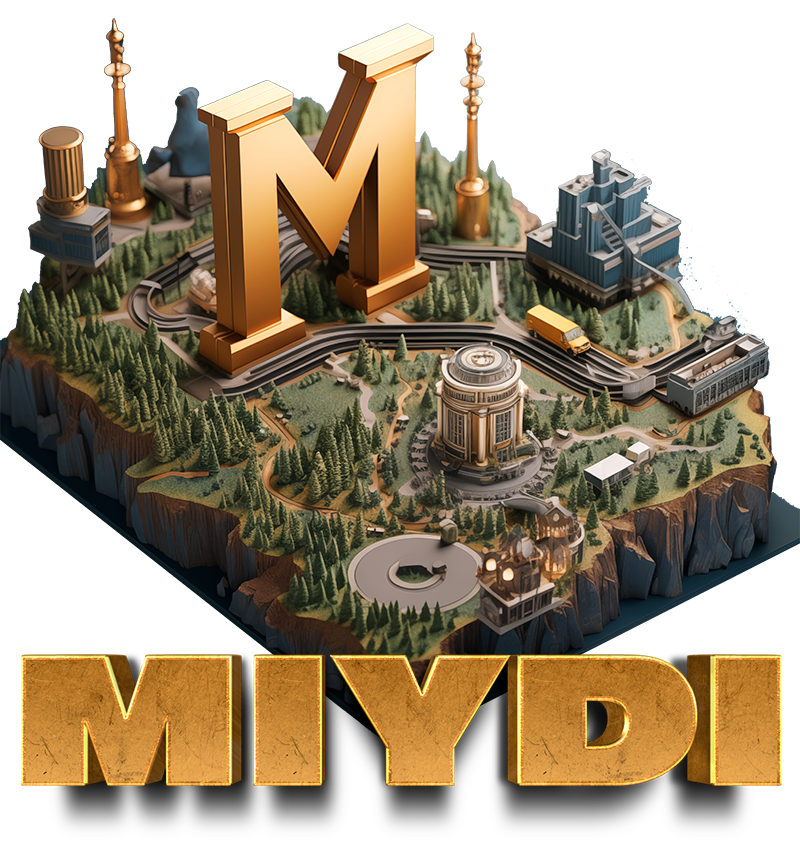
Miydi Maps is an extensive repository dedicated to providing highly detailed socio-economic data, meticulously compiled down to individual census tracts and zip codes. This initiative is designed to streamline the utilization of geographic data across various advanced applications, including Artificial Intelligence, data analytics, urban planning, and more, by eliminating the complexities of data parsing.
Introducing Miydi Maps
Free AI Optimized Geo-Spatial Data Repo
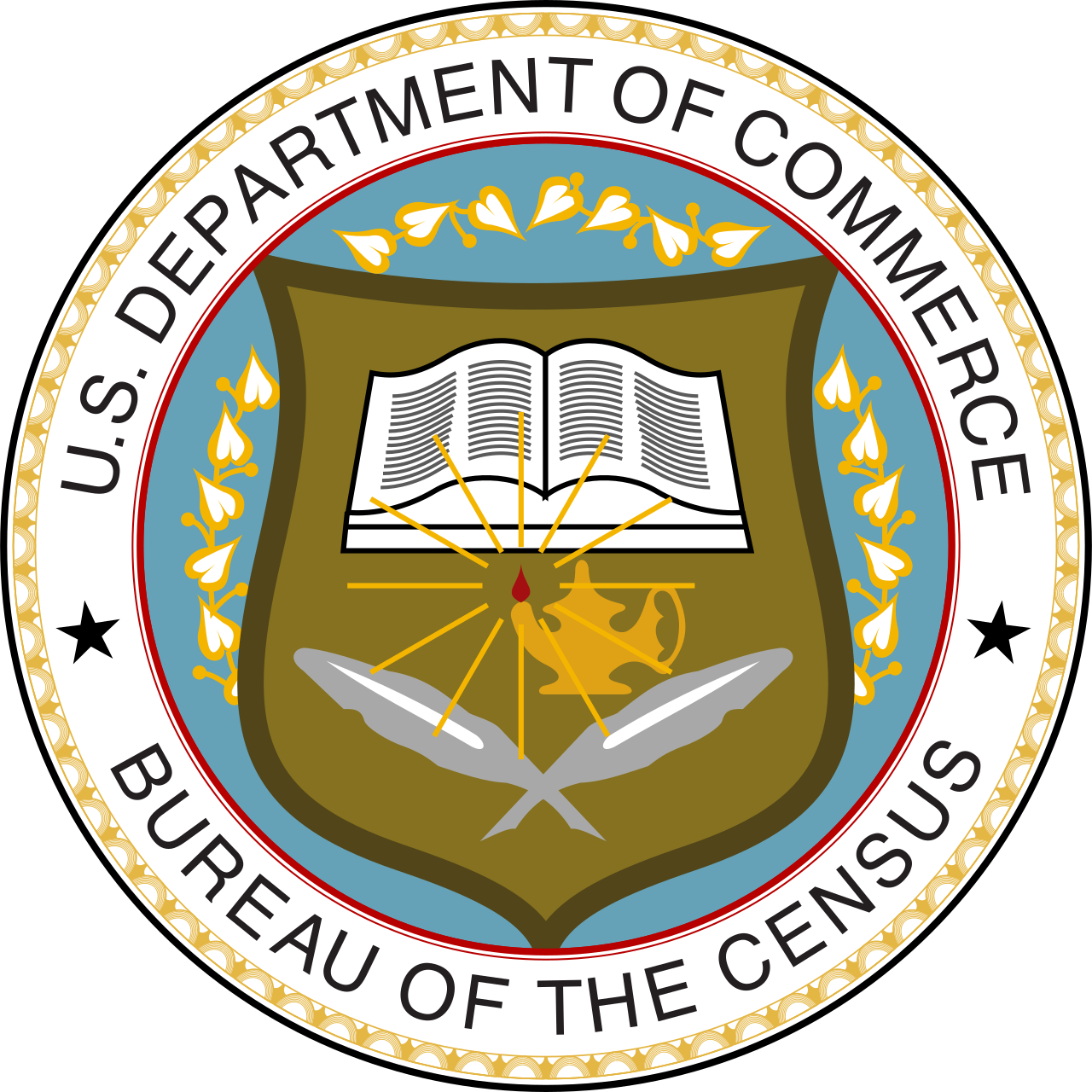
Miydi Maps is a valuable open-source geospatial resource available on GitHub, offering detailed integration of optimized OpenStreetMap (OSM) data with extensive demographic information down to the census tract level. This resource combines data from various government and some private sector sources, making it a highly useful tool for urban planners, researchers, and policy makers who need precise, localized geographic analyses.
Free access to such comprehensive data supports a wide range of users, encouraging collaboration and innovation across different fields. Miydi Maps not only provides robust and accurate geographic data but also ensures easy usability with mapping tools like QGIS. This accessibility allows users to create in-depth, data-driven geospatial visualizations and analyses effortlessly.
Census Tracts
Miydi Maps starts with Census tracts as its foundational layer, enabling detailed mapping and analysis. This setup allows the integration of diverse demographic data, enhancing granularity and utility.
Additionally, a robust connection layer in Miydi Maps facilitates the seamless linking of various demographic datasets to specific Census tracts. This flexibility supports a wide range of analytical and planning needs, making it a versatile tool for mapping projects.
Roads and Neighborhoods
Miydi Maps incorporates detailed land use data specifically for residential areas, mapping out various types of housing and land allocation. This layer is crucial for understanding the spatial distribution and characteristics of living spaces within different regions. It provides a clear visualization of residential zones, helping users analyze housing patterns and urban development.
In addition to land use data, Miydi Maps integrates OpenStreetMap (OSM) neighborhood maps to offer a more nuanced view of community layouts. This combination enriches the dataset, allowing for a deeper insight into how neighborhoods are structured and interconnected. It also enhances the ability to assess amenities, services, and the overall livability of different areas.
The mapping solution is further refined by the inclusion of detailed street and road maps. These maps provide essential information on the transportation networks that connect residential areas to the broader urban infrastructure. The detailed road data helps in planning routes, understanding traffic flow, and exploring connectivity between different parts of the city or region.
Land Use
Miydi Maps extends its capabilities to encompass retail and business areas, meticulously tracking them by zoning and land use categories. This integration allows users to visualize commercial landscapes, identify business clusters, and analyze economic activities within specific zones. By aligning detailed zoning information with business types, Miydi Maps provides a valuable tool for urban planners and business analysts to strategize and optimize commercial development.
Further enhancing its utility, Miydi Maps includes optimized OpenStreetMap (OSM) layers specifically for commercial buildings. These layers categorize buildings by type, such as retail stores, food services, and bars, presented in both multipolygon and centroid formats. This approach supports diverse mapping needs, from high-level strategic planning to detailed local area studies, enabling precise visualization and analysis of commercial structures.
Additionally, the platform incorporates essential city services and cultural sites within its datasets. Locations such as hospitals, schools, and churches are mapped, offering a comprehensive view of community infrastructure and amenities. This data is crucial for a variety of applications, including emergency planning, cultural research, and community services development, making Miydi Maps an indispensable resource for a broad spectrum of users.
Has a scale for individual buildings (1:1 scale)
Miydi Maps delivers granular details down to individual buildings, cataloging each structure’s purpose or function across residential, government, commercial, and industrial categories.
Additionally, this building-specific data is fully integrable with census-level demographic data, enriching the geographic and demographic understanding of any locality.
Simplified AI View
Miydi Maps is meticulously optimized for GeoSpatial AI applications, ensuring that the data is structured in a way that maximizes usability for artificial intelligence systems.
By organizing the extensive demographic and census data into distinct buckets, the platform facilitates easier access and manipulation by AI algorithms.
This bucketing method simplifies the process of querying and analyzing complex datasets, allowing AI models to efficiently process and interpret spatial and demographic patterns. The strategic structuring of data not only accelerates computational tasks but also improves the accuracy and relevancy of AI-driven insights.
This refined data organization enhances the AI’s capability to reference specific datasets quickly or display detailed demographic information in a user-friendly manner. By streamlining how data is presented and accessed, Miydi Maps makes it feasible for AI technologies to generate sophisticated visualizations and predictive analyses.
Free and Open Source
Miydi Maps stands out as an open-source treasure trove of geospatial data, freely accessible to anyone interested in demographic or census-related visualizations.
This accessibility democratizes the availability of high-quality geographic information, enabling users to launch projects without the constraints of licensing fees or proprietary software.
Build your own GeoSpatial AI Applications
Endlessly combine Data Sets
Miydi Maps is fully customizable, allowing users to tailor any type of visualization to meet their specific needs. We encourage all users to explore the extensive documentation available on GitHub, which provides detailed guidance on how to maximize the potential of the datasets and tools provided.
By downloading Miydi Maps for yourself, you can experiment with and adapt the data, unlocking new possibilities for visualizing and analyzing geographic and demographic information. Dive into the resources and start crafting your unique visualizations today!
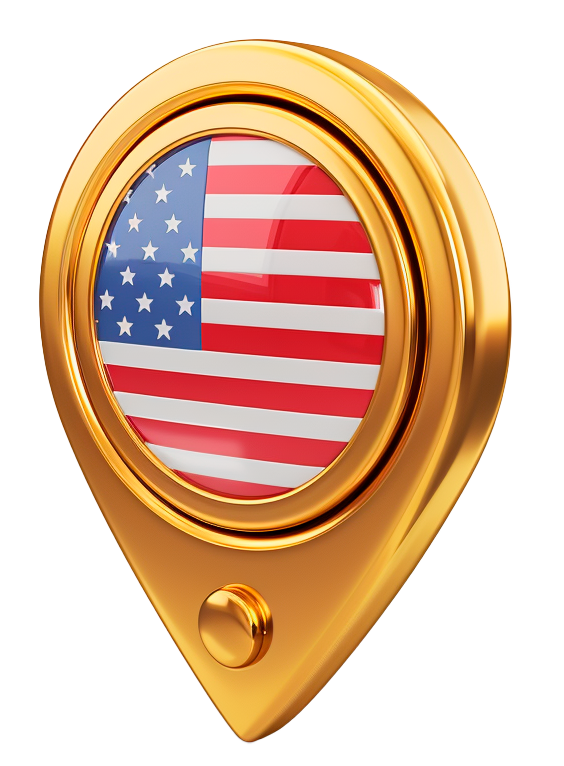
Compiled from official US Government Data
We gathered and consolidated datasets from key U.S. government agencies, including the Census Bureau, IRS, CDC, and NASA, as well as extensive resources on Data.gov.
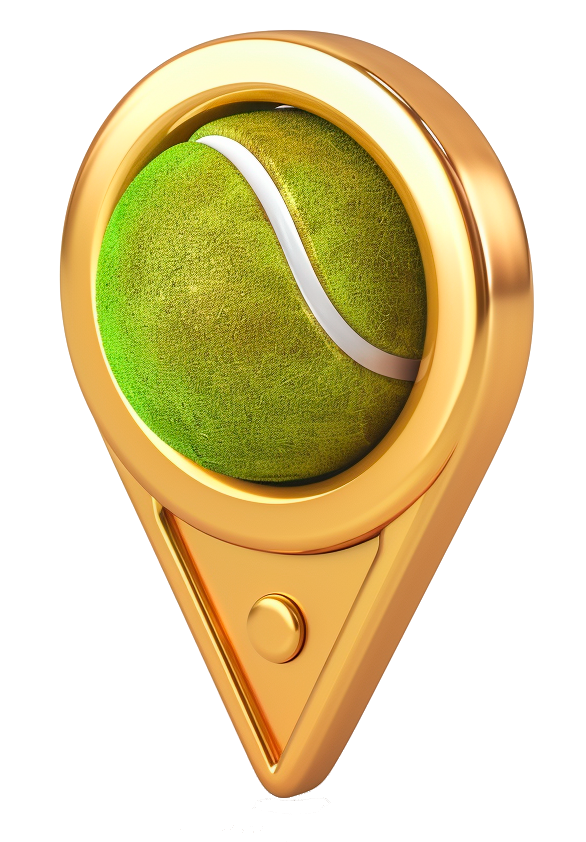
Lifestyle and Recreation Data
By leveraging OpenStreetMap (OSM) data, we can catalog local recreation spots, lifestyle amenities, and sporting venues in any given area. This information is then correlated with the demographic data to construct a clear, user-friendly model of the population’s habits and preferences.
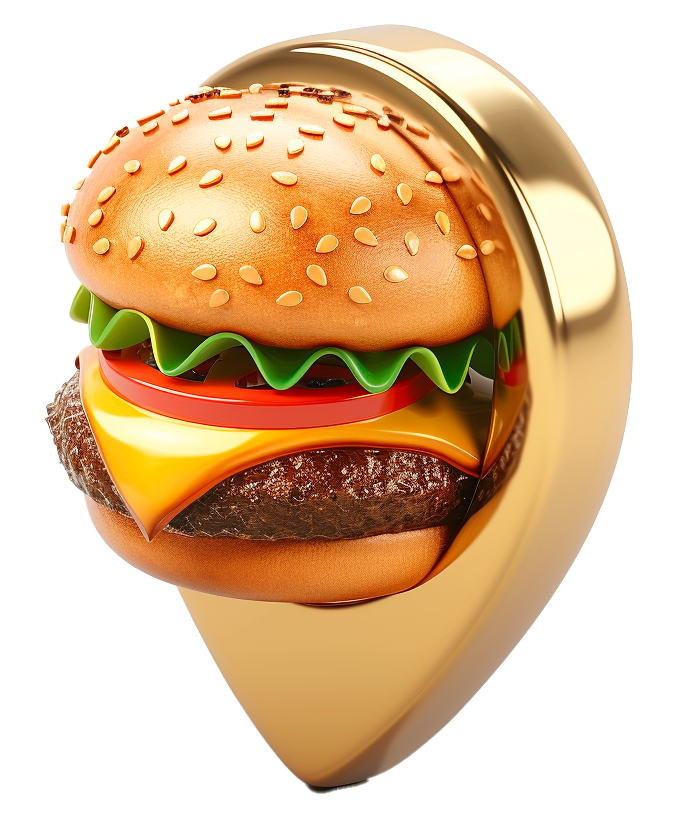
Retail and Commercial Activity
We integrate OpenStreetMap (OSM) data on retail and commercial establishments—encompassing shops, restaurants, office buildings, and financial institutions. This correlation with demographic data enables the creation of a detailed model illustrating where economic activities cluster, how they evolve over time, and which factors drive local commerce and growth.
Compiling the data
Data then optimized for AI applications
Miydi Maps prioritizes data optimization for AI by breaking down complex datasets into their smallest meaningful components. By dismantling large aggregates into discrete, easily identifiable units, we ensure that machine learning models can process each piece of information individually and draw more accurate, context-specific conclusions.
Each data point is then placed in an isolated “bucket,” simplifying organization and retrieval across various AI workflows. This structured approach not only speeds up processing times, but also aids in data filtering, validation, and enhancement, ultimately improving the quality and reliability of AI-driven insights.
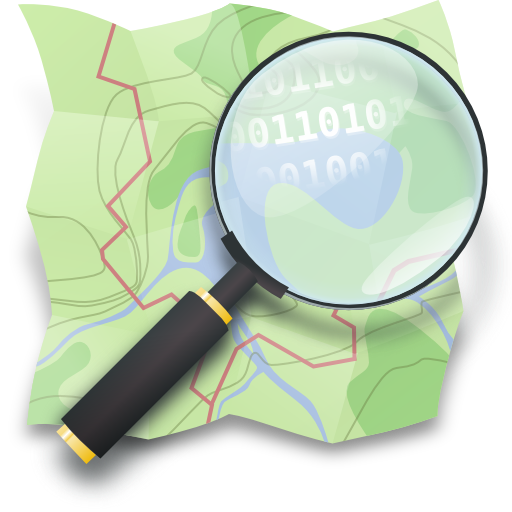
Based around ITIL
We organize our datasets following ITIL principles to ensure consistent, standardized processes and clear documentation. By aligning data handling with ITIL’s structured approach, we maintain high-quality records that are easily traceable, reducing errors and promoting reliable, repeatable outcomes.
Documented with KCS
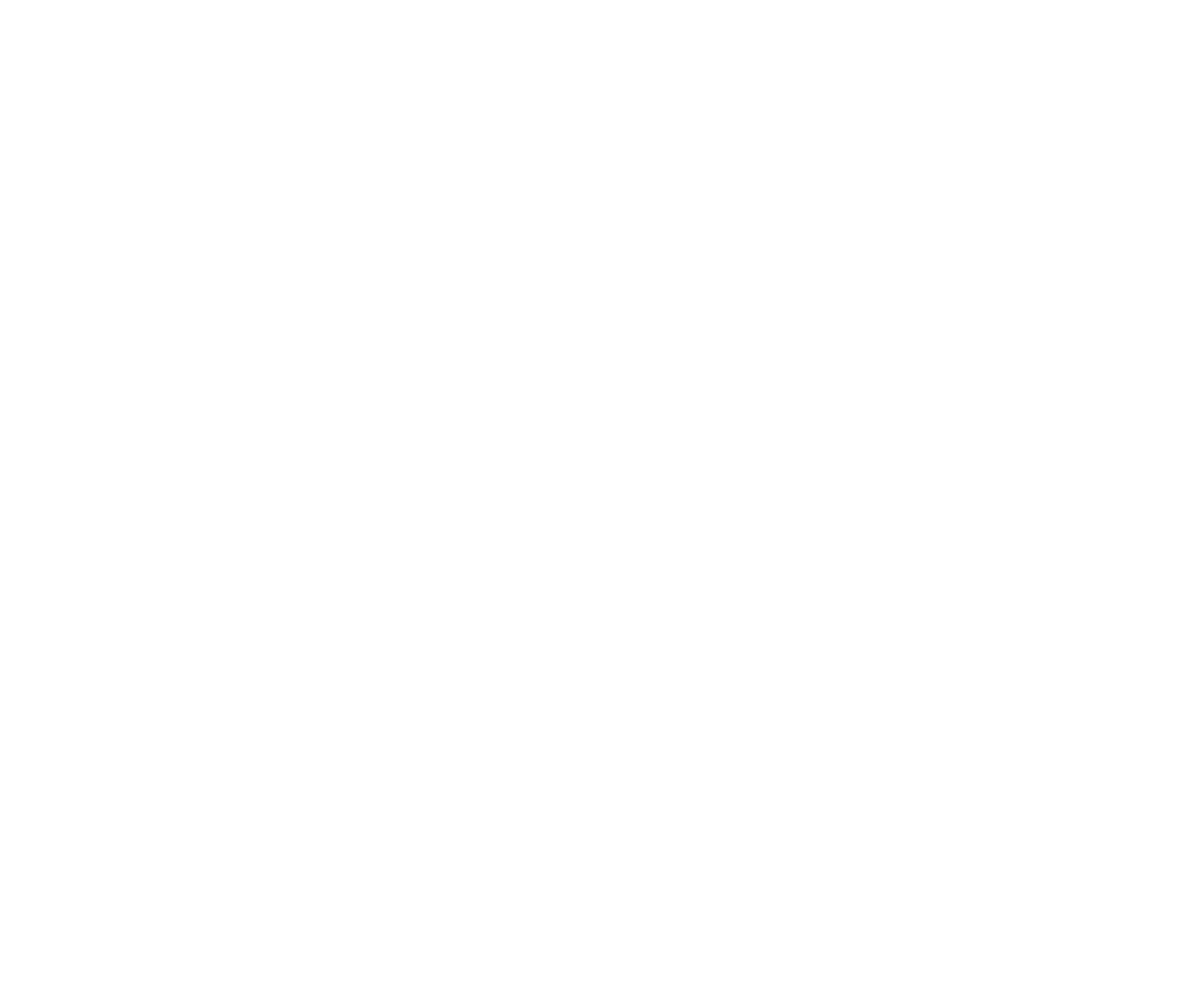
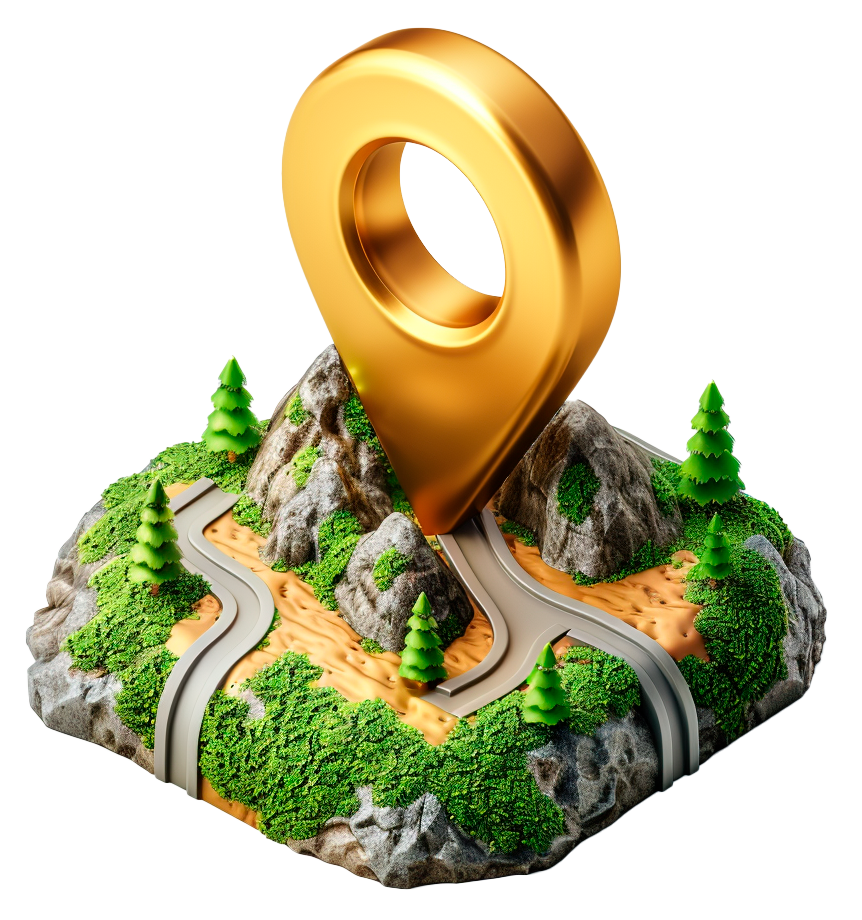